Piecewise polynomials and splines#
1D interpolation routines discussed in the previous section, work by constructing certain piecewise polynomials: the interpolation range is split into intervals by the so-called breakpoints, and there is a certain polynomial on each interval. These polynomial pieces then match at the breakpoints with a predefined smoothness: the second derivatives for cubic splines, the first derivatives for monotone interpolants and so on.
A polynomial of degree \(k\) can be thought of as a linear combination of
\(k+1\) monomial basis elements, \(1, x, x^2, \cdots, x^k\).
In some applications, it is useful to consider alternative (if formally
equivalent) bases. Two popular bases, implemented in scipy.interpolate
are
B-splines (BSpline
) and Bernstein polynomials (BPoly
).
B-splines are often used for, for example, non-parametric regression problems,
and Bernstein polynomials are used for constructing Bezier curves.
PPoly
objects represent piecewise polynomials in the ‘usual’ power basis.
This is the case for CubicSpline
instances and monotone interpolants.
In general, PPoly
objects can represent polynomials of
arbitrary orders, not only cubics. For the data array x
, breakpoints are at
the data points, and the array of coefficients, c
, define polynomials of
degree \(k\), such that c[i, j]
is a coefficient for
(x - x[j])**(k-i)
on the segment between x[j]
and x[j+1]
.
BSpline
objects represent B-spline functions — linear combinations of
b-spline basis elements.
These objects can be instantiated directly or constructed from data with the
make_interp_spline
factory function.
Finally, Bernstein polynomials are represented as instances of the BPoly
class.
All these classes implement a (mostly) similar interface, PPoly
being the most
feature-complete. We next consider the main features of this interface and
discuss some details of the alternative bases for piecewise polynomials.
Manipulating PPoly
objects#
PPoly
objects have convenient methods for constructing derivatives
and antiderivatives, computing integrals and root-finding. For example, we
tabulate the sine function and find the roots of its derivative.
>>> import numpy as np
>>> from scipy.interpolate import CubicSpline
>>> x = np.linspace(0, 10, 71)
>>> y = np.sin(x)
>>> spl = CubicSpline(x, y)
Now, differentiate the spline:
>>> dspl = spl.derivative()
Here dspl
is a PPoly
instance which represents a polynomial approximation
to the derivative of the original object, spl
. Evaluating dspl
at a
fixed argument is equivalent to evaluating the original spline with the nu=1
argument:
>>> dspl(1.1), spl(1.1, nu=1)
(0.45361436, 0.45361436)
Note that the second form above evaluates the derivative in place, while with
the dspl
object, we can find the zeros of the derivative of spl
:
>>> dspl.roots() / np.pi
array([-0.45480801, 0.50000034, 1.50000099, 2.5000016 , 3.46249993])
This agrees well with roots \(\pi/2 + \pi\,n\) of \(\cos(x) = \sin'(x)\). Note that by default it computed the roots extrapolated to the outside of the interpolation interval \(0 \leqslant x \leqslant 10\), and that the extrapolated results (the first and last values) are much less accurate. We can switch off the extrapolation and limit the root-finding to the interpolation interval:
>>> dspl.roots(extrapolate=False) / np.pi
array([0.50000034, 1.50000099, 2.5000016])
In fact, the root
method is a special case of a more general solve
method which finds for a given constant \(y\) the solutions of the
equation \(f(x) = y\) , where \(f(x)\) is the piecewise polynomial:
>>> dspl.solve(0.5, extrapolate=False) / np.pi
array([0.33332755, 1.66667195, 2.3333271])
which agrees well with the expected values of \(\pm\arccos(1/2) + 2\pi\,n\).
Integrals of piecewise polynomials can be computed using the .integrate
method which accepts the lower and the upper limits of integration. As an
example, we compute an approximation to the complete elliptic integral
\(K(m) = \int_0^{\pi/2} [1 - m\sin^2 x]^{-1/2} dx\):
>>> from scipy.special import ellipk
>>> m = 0.5
>>> ellipk(m)
1.8540746773013719
To this end, we tabulate the integrand and interpolate it using the monotone
PCHIP interpolant (we could as well used a CubicSpline
):
>>> from scipy.interpolate import PchipInterpolator
>>> x = np.linspace(0, np.pi/2, 70)
>>> y = (1 - m*np.sin(x)**2)**(-1/2)
>>> spl = PchipInterpolator(x, y)
and integrate
>>> spl.integrate(0, np.pi/2)
1.854074674965991
which is indeed close to the value computed by scipy.special.ellipk
.
All piecewise polynomials can be constructed with N-dimensional y
values.
If y.ndim > 1
, it is understood as a stack of 1D y
values, which are
arranged along the interpolation axis (with the default value of 0).
The latter is specified via the axis
argument, and the invariant is that
len(x) == y.shape[axis]
. As an example, we extend the elliptic integral
example above to compute the approximation for a range of m
values, using
the NumPy broadcasting:
>>> from scipy.interpolate import PchipInterpolator
>>> m = np.linspace(0, 0.9, 11)
>>> x = np.linspace(0, np.pi/2, 70)
>>> y = 1 / np.sqrt(1 - m[:, None]*np.sin(x)**2)
Now the y
array has the shape (11, 70)
, so that the values of y
for fixed value of m
are along the second axis of the y
array.
>>> spl = PchipInterpolator(x, y, axis=1) # the default is axis=0
>>> import matplotlib.pyplot as plt
>>> plt.plot(m, spl.integrate(0, np.pi/2), '--')
>>> from scipy.special import ellipk
>>> plt.plot(m, ellipk(m), 'o')
>>> plt.legend(['`ellipk`', 'integrated piecewise polynomial'])
>>> plt.show()
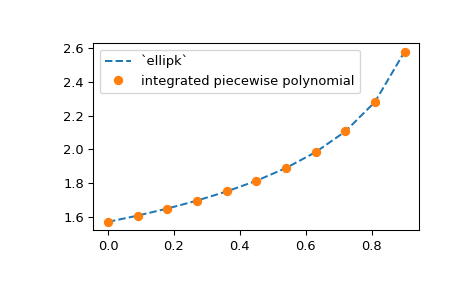
B-splines: knots and coefficients#
A b-spline function — for instance, constructed from data via a
make_interp_spline
call — is defined by the so-called knots and coefficients.
As an illustration, let us again construct the interpolation of a sine function.
The knots are available as the t
attribute of a BSpline
instance:
>>> x = np.linspace(0, 3/2, 7)
>>> y = np.sin(np.pi*x)
>>> from scipy.interpolate import make_interp_spline
>>> bspl = make_interp_spline(x, y, k=3)
>>> print(bspl.t)
[0. 0. 0. 0. 0.5 0.75 1. 1.5 1.5 1.5 1.5 ]
>>> print(x)
[ 0. 0.25 0.5 0.75 1. 1.25 1.5 ]
We see that the knot vector by default is constructed from the input
array x
: first, it is made \((k+1)\) -regular (it has k
repeated knots appended and prepended); then, the second and
second-to-last points of the input array are removed—this is the so-called
not-a-knot boundary condition.
In general, an interpolating spline of degree k
needs
len(t) - len(x) - k - 1
boundary conditions. For cubic splines with
(k+1)
-regular knot arrays this means two boundary conditions—or
removing two values from the x
array. Various boundary conditions can be
requested using the optional bc_type
argument of make_interp_spline
.
The b-spline coefficients are accessed via the c
attribute of a BSpline
object:
>>> len(bspl.c)
7
The convention is that for len(t)
knots there are len(t) - k - 1
coefficients. Some routines (see the Smoothing splines section) zero-pad the c
arrays so that
len(c) == len(t)
. These additional coefficients are ignored for evaluation.
We stress that the coefficients are given in the b-spline basis, not the power basis of \(1, x, \cdots, x^k\).
B-spline basis elements#
B-splines are piecewise polynomials, represented as linear combinations of b-spline basis elements — which themselves are certain linear combinations of usual monomials, \(x^m\) with \(m=0, 1, \dots, k\).
The b-spline basis is generally more computationally stable than the power basis and is useful for a variety of applications which include interpolation, regression and curve representation. The main feature is that these basis elements are localized and equal to zero outside of an interval defined by the knot array.
Specifically, a b-spline basis element of degree k
(e.g. k=3
for cubics)
is defined by \(k+2\) knots and is zero outside of these knots.
To illustrate, plot a collection of non-zero basis elements on a certain
interval:
>>> k = 3 # cubic splines
>>> t = [0., 1.4, 2., 3.1, 5.] # internal knots
>>> t = np.r_[[0]*k, t, [5]*k] # add boundary knots
>>> from scipy.interpolate import BSpline
>>> import matplotlib.pyplot as plt
>>> for j in [-2, -1, 0, 1, 2]:
... a, b = t[k+j], t[-k+j-1]
... xx = np.linspace(a, b, 101)
... bspl = BSpline.basis_element(t[k+j:-k+j])
... plt.plot(xx, bspl(xx), label=f'j = {j}')
>>> plt.legend(loc='best')
>>> plt.show()
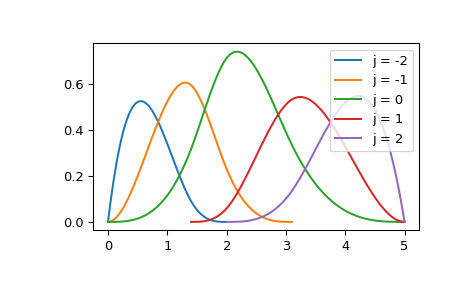
Here BSpline.basis_element
is essentially a shorthand for constructing a spline
with only a single non-zero coefficient. For instance, the j=2
element in
the above example is equivalent to
>>> c = np.zeros(t.size - k - 1)
>>> c[-2] = 1
>>> b = BSpline(t, c, k)
>>> np.allclose(b(xx), bspl(xx))
True
If desired, a b-spline can be converted into a PPoly
object using
PPoly.from_spline
method which accepts a BSpline
instance and returns a
PPoly
instance. The reverse conversion is performed by the
BSpline.from_power_basis
method. However, conversions between bases is best
avoided because it accumulates rounding errors.
Design matrices in the B-spline basis#
One common application of b-splines is in non-parametric regression. The reason is that the localized nature of the b-spline basis elements makes linear algebra banded. This is because at most \(k+1\) basis elements are non-zero at a given evaluation point, thus a design matrix built on b-splines has at most \(k+1\) diagonals.
As an illustration, we consider a toy example. Suppose our data are
one-dimensional and are confined to an interval \([0, 6]\).
We construct a 4-regular knot vector which corresponds to 7 data points and
cubic, k=3
, splines:
>>> t = [0., 0., 0., 0., 2., 3., 4., 6., 6., 6., 6.]
Next, take ‘observations’ to be
>>> xnew = [1, 2, 3]
and construct the design matrix in the sparse CSR format
>>> from scipy.interpolate import BSpline
>>> mat = BSpline.design_matrix(xnew, t, k=3)
>>> mat
<Compressed Sparse Row sparse array of dtype 'float64'
with 12 stored elements and shape (3, 7)>
Here each row of the design matrix corresponds to a value in the xnew
array,
and a row has no more than k+1 = 4
non-zero elements; row j
contains basis elements evaluated at xnew[j]
:
>>> with np.printoptions(precision=3):
... print(mat.toarray())
[[0.125 0.514 0.319 0.042 0. 0. 0. ]
[0. 0.111 0.556 0.333 0. 0. 0. ]
[0. 0. 0.125 0.75 0.125 0. 0. ]]